Unlocking the power of computational tools in protein complex prediction
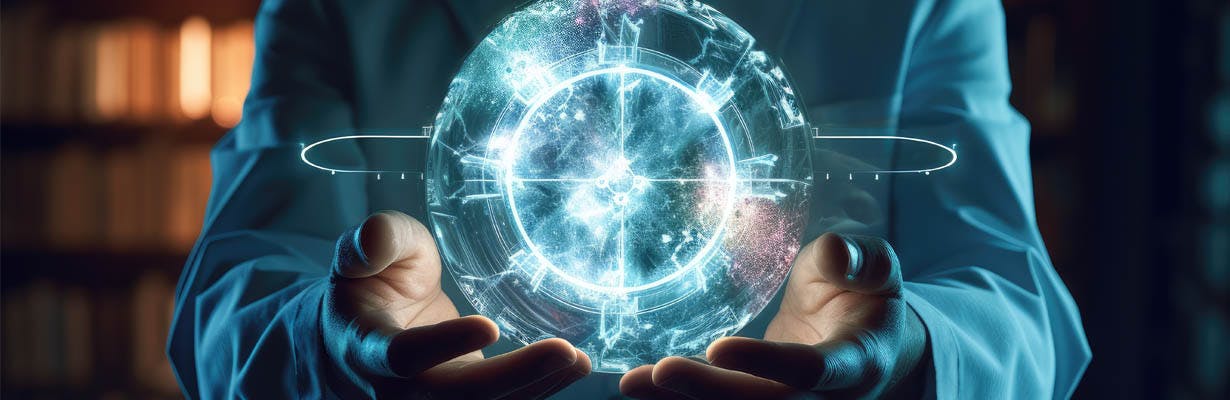
Protein complexes are essential units that drive cellular functions, formed by intricate protein-protein interactions (PPIs). Despite advancements in experimental methods, identifying the vast array of protein complexes — termed the complexome — remains a significant challenge. Computational tools have become pivotal in bridging the gap, leveraging both PPI data and structural biology.
The complex landscape of protein interactions
Proteins rarely function in isolation. Interactions between thousands of proteins shape a cell’s PPI network, forming transient or stable complexes. Experimental techniques such as mass spectrometry, yeast two-hybrid assays, and structural analyses (e.g., NMR spectroscopy) have laid the foundation for understanding PPIs. However, these methods often lack scalability or the resolution needed for a holistic view of the complexome.
This is where computational approaches shine. They harness machine learning (ML), simulations, and structural data to predict complex compositions, structures, and even dynamics under varying biological conditions.
Computational approaches in focus
- Structure-based predictions
- Deep learning models: AlphaFold-Multimer and similar tools have revolutionized the field by predicting protein complex structures with remarkable accuracy. They utilize amino acid sequences to generate 3D models, extending beyond monomeric predictions to heteromeric and homomeric complexes.
- Docking simulations: Traditional tools like ZDOCK and ClusPro simulate molecular interactions at the atomic level. While computationally intensive, these models provide detailed insights into binding interfaces.
- PPI network clustering
- Graph-Based methods: Algorithms like Markov Clustering (MCL) and ClusterOne analyze dense subgraphs within PPI networks to identify potential complexes. These methods scale well to predict the entire complexome but may lack fine-grained structural details.
- Generative models: Tools like PcGAN use adversarial networks to generate plausible protein complexes, uncovering novel associations that may evade traditional clustering.
- Hybrid simulations
- Combining rule-based modeling with experimental data, simulations predict not only the formation of complexes but also their abundance and variations across conditions. For example, Cytocast's simulations provide quantitative insights into how perturbations — like mutations, diseases or drug effects — ripple through the complexome.
Future directions and challenges
The ultimate goal of computational protein complex prediction is to illuminate how these complexes govern cellular behavior and to harness this understanding for therapeutic advancements. Emerging trends include:
- Dynamic modeling: Transitioning from static predictions to models that simulate the dynamic assembly and disassembly of complexes.
- Integration of phase separation: Incorporating the phenomenon of protein phase separation, which impacts cellular compartmentalization and interactions.
- Post-translational modifications: Linking modifications such as phosphorylation to changes in PPI networks and complex stability.
The convergence of high-throughput experimental techniques, deep learning, and enhanced computational power promises a future where even subtle changes in a single protein can be mapped across the complexome, unveiling new dimensions of cellular regulation.
Cytocast: pioneering quantitative predictions
Cytocast's proprietary tools exemplify the cutting-edge integration of PPI simulations with real-world biological data. By offering quantitative and context-specific predictions, Cytocast empowers researchers to delve deeper into the dynamic interplay of proteins, setting a benchmark for the future of computational biology.
In the ever-evolving landscape of structural biology, computational tools are not just aids but essential drivers, transforming how we understand and manipulate the molecular machinery of life.
Read our previous blogpost: Virtual pathways to cures: the future of cellular modeling in pharmaceuticals